Digital transformation marches on, and with it, the volume of data generated by your organization grows exponentially. As your organization embraces more cloud, container, edge computing, and ephemeral services, the ability to maintain control of data is out of reach of your current tools—despite the universal knowledge that data is one of the most valuable assets for your organization.
The term “shadow data” and “shadow databases” has been used widely by some industry analysts and other enterprises working in the data security space to describe any data that is unknown, unmanaged, or undiscovered by data discovery tools like data security posture management (DSPM). While the term “shadow data” continues to enjoy some marketing popularity among a few, it has also created greater confusion and unnecessary fear, uncertainty, and doubt (FUD) by falsely oversimplifying the challenge with the various forms of unknown, unmanaged, or undiscovered data.
So What’s Wrong With Using the Term Shadow Data?
While the simplicity and marketing bang of the term is undeniable, using a term like this does an incredible disservice to data security efforts. First, by shoe-horning the various forms of unknown, unmanaged, or undiscovered data under one broad, overgeneralized topic, it convolutes how organizations need to approach data security efforts. In addition, the term ‘shadow data’ suggests that data is inherently dangerous or nefarious. It implies that this data is lurking in the shadows, waiting to cause harm. As we described in Treat Data Like Your Family, data on its own doesn’t become more dangerous, but can become so based on the actions, attitudes, and sometimes inaction of others. The FUD created by this term is so strong that no-one actually knows what it means, they are just looking for a solution to find their shadow data. We might as well just say “data that should not be named.”
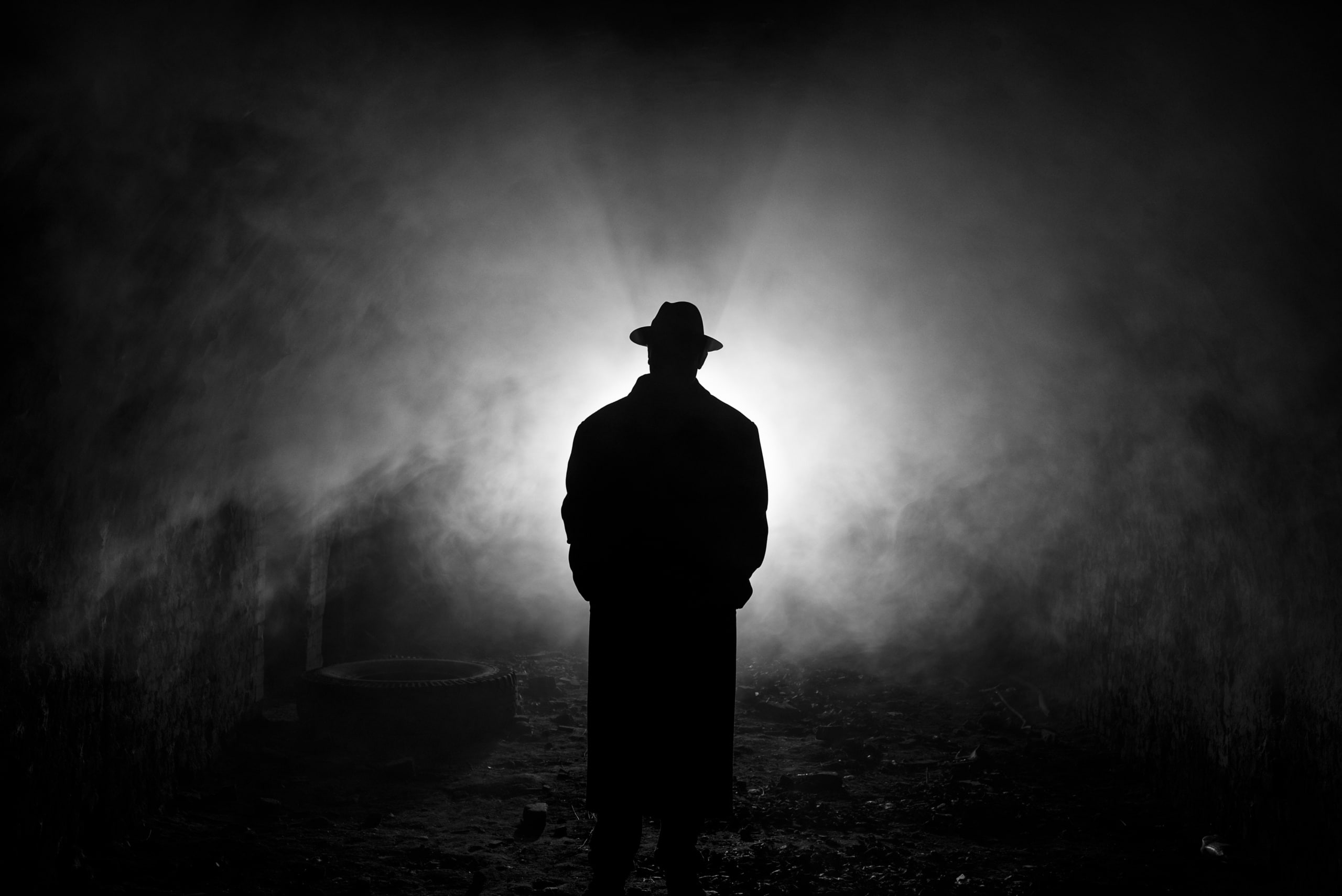
The current and exceedingly generalized ‘definition’ of “shadow” data can include numerous, broad data types, such as:
- Data that we (usually in the security context) didn’t know we stored.
- Data that an organization no longer needs or uses, or worse never needed.
- Data that an individual contributor (i.e. a developer or analyst) is working on, but exists outside the purview of the security and data governance teams.
- Data stored on personal devices.
- Data stored in embedded memory.
- Data in SaaS services, including ChatGPT.
- Data in other non-sanctioned physical locations, such as a local MySQL database hosted in a forgotten cupboard.
The “shadows” of each of these are obviously completely different and require different processes and sometimes tools to shine a light on and manage. This perception, by grouping all of this into a single term that a single product solves, is not only inaccurate but also unhelpful in managing data effectively. Instead, as an industry we should focus on describing shadow data more accurately, using terms that are descriptive and informative.
Let’s Get More Descriptive
To better describe “shadow” data, we propose the use of more descriptive terms. These terms provide a more accurate and nuanced view, allowing organizations to better understand their data landscape and make informed decisions about how to address the issues with the data.
- Redundant data refers to data that is duplicated across multiple locations, often without a clear reason. The storage of redundant data is unnecessary and organizations should implement processes for deleting it.
- Obsolete data refers to data that is no longer relevant or useful, for example, data about discontinued products. This type of data can be a liability, taking up storage space and unnecessarily increasing the blast radius of security incidents. For obsolete data, organizations should implement processes for deleting or archiving it in accordance with compliance requirements, particularly around legal requirements for retention.
- Dormant data refers to data that has not been accessed or used in a long time. This type of data may still be useful but is not currently being used. Organizations should develop processes for moving dormant data stores to lower-cost storage tiers, restricting unused access or implementing policies for automatically archiving dormant data after a certain period of time.
- Trivial data refers to data that is of little or no value to the organization. This type of data can be a liability, taking up valuable storage space and potentially leading to compliance issues if not properly managed, for example, the storage of copyrighted video within a corporate environment.
- Unmanaged data stores refer to locations where data is stored outside of managed data stores. The storage of data in these locations can be security risks and a compliance liability, as it is often not subject to the same controls as managed data stores. This can include:
- Embedded databases that are hidden within other hardware or software. This type of data can be hard to find and manage outside, making it a potential security risk.
- SaaS services including personal storage.
- Misplaced data refers to data that is stored in the wrong location or not properly classified. This type of data can be hard to find and manage, leading to potential compliance issues and security risks. Organizations should develop processes to continuously identify and classify data in all their environments and control data sprawl between these environments.

By accurately describing unmanaged data, organizations can better understand their data and leave nothing in the shadows, ultimately facilitating better and more informed decision-making about how to manage data. In addition, organizations should implement a range of approaches and tools, including data classification, deletion, migration, archiving, discovery, and classification. By adopting these approaches and tools, businesses can reduce the risks associated with shadow data, improve compliance, and make better use of their valuable data assets.
While there is no magic formula for identifying all the various forms of shadow data, a data security posture management (DSPM) solution can be an immense help in understanding and prioritizing your data within your hybrid cloud. Other tools that are useful for discovering shadow data include modern CASB and DLP tools to discover data flowing to SaaS tools and stored on physical endpoints, and network discovery tools to find unknown data stores in your on-premise environments.
To learn more about DSPM or see a DSPM solution in action, please reach out. We’d love to show you how Symmetry DataGuard can help drastically improve the number of dormant identities and the length of their dormancy.